Robotic autonomy in endocrine surgery
Introduction
Novel robotic techniques and instrumentation are changing our approach to many surgical procedures. The surgical robot is now a familiar tool, seen in many operating rooms across the globe. In the realm of endocrine surgery, parathyroid and thyroid surgery remain most commonly performed in an open fashion. Still, over the past decade, we have witnessed an expansion of robotic surgery into head and neck endocrine surgery. Robotic thyroidectomy has been described using the retroauricular or facelift approach (1,2), transaxillary approach (3-5), bilateral axillo-breast approach (6,7) and transoral approach (8-10). Though not widely practiced, some institutions have gained extensive experience with robotic endocrine procedures (5,11).
Surgical robotic technologies are in a constant state of evolution. Innovative alterations are driving toward instrument diversification and miniaturization, enhanced navigation, improved haptic and visual feedback, as well as increased flexibility and maneuverability for nonlinear access. Another area of innovation, which remains largely in the experimental stage, is the development of autonomous robotic functions. We are in an era where robotic autonomy is commonplace outside of operating rooms. Starkly contrasted with the autonomous vehicles that are becoming common on our roads, robotic endocrine surgery of today relies entirely on direct surgeon control.
Fully autonomous robotic surgery, in which a robot is able to perform surgery without human intervention, still resides in the realm of science fiction. However, the implementation of robotic autonomy to enhance our capabilities is currently being explored. Herein, we will provide a framework for understanding surgical robotic autonomy and discuss some ways that autonomy may be applied to endocrine surgery in the future.
Spectrum of robotic autonomy
Fully autonomous surgery involves the performance of surgical tasks by a robotic system, without human intervention. Robotic autonomy exists on a spectrum, with a variable degree of robotic and surgeon involvement. Four categories are described to classify automation: direct control, shared control, supervisory control, and full autonomy. It is important to keep in mind that a robotic platform may perform functions from more than one category (12).
In direct control systems, the user is in full control of the robotic functions with the robot mirroring the surgeon’s movements without any automated assistance. The da Vinci Surgical System (Intuitive Surgical, Sunnyvale, CA) is an example of a direct control system. The human surgeon is in complete control of robotic actions.
In shared control systems, the robot and the surgeon share command of the instrument manipulators and work together. Rather than directly mirroring the surgeon’s movements, the robot may apply tremor reduction or motion scaling. An example of this is the Steady Hand Robot (Johns Hopkins University, Baltimore, MD), which applies counteractive forces proportional to the force sensed by the instrument to enable fine motion and precision for sub-millimeter surgical tasks (13,14).
In supervisory control systems, the surgeon is in charge of decision-making and surgical planning, but the robot executes the task. This decouples the commands of the surgeon from the actions of the robot. An example of supervisory control is stereotactic radiosurgery (e.g., CyberKnife), in which the surgeon adjusts a computer-generated plan prior to execution to ensure the system performs the task safely and effectively (15).
The final category is full autonomy, where a robotic system replaces the intraoperative role of a human surgeon. Such a system would have sophisticated sensory capabilities and the ability to plan and execute entire operations. This category exists in theory and is not likely to be realized in practice in the foreseeable future (12).
Surgical robotic autonomy
In order to perform an automated surgical task or procedure, a robot must be able to sense, plan, and act (Figure 1). We will develop these three capacities, explore what they involve, and discuss their importance (12).
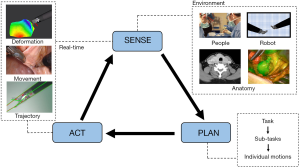
Sensing refers to the ability to know the surgical environment. Sensory information defines what is in the surgical field, where it is in space, and where each component is in relation to other structures. This means knowing the contour, composition and characteristics of the operative field and tissue. It also means knowing the environment around the tissue and surgical drapes, patient vital signs, and the robotic instrument location and motion paths. A surgeon seamlessly integrates visual information, spatial awareness, and tactile feedback with an intimate understanding of human anatomy. To mimic this degree of highly sophisticated perception and processing, an autonomous robot would need to continuously collect and process visual data to determine the position, orientation, depth and texture of each component of the surgical field. The system must also have a way to collect information about the environment deep to the visible topography of the field as surgery is rarely performed in a single plane.
Current semi-autonomous robotic platforms utilize three-dimensional reconstructions created from preoperative imaging that are registered to the patient intraoperatively as a manner of “sensing” the operative environment. However, this is not real-time sensing, and can only be used in static environments like bony anatomy where the preoperative model is identical to the anatomy of the surgical field (see below). This preoperative imaging method cannot be applied to a soft tissue environment, as tissues are deformable and may not be identical (in location, space, size or character) to a static preoperative scan. In soft tissue environments, sensory data collection has been performed in the research setting using real-time ultrasound (12), instrument tracking methods (16), and fluorescent technologies (17).
Developing a technology that can accurately and rapidly sense and analyze the complexities of a dynamic surgical field is extremely challenging. Unlike bony anatomy of orthopedic or skull base surgery, the soft tissues of the neck are deformable, constantly changing throughout an operation. Every time tissue is retracted, dissected, divided or cauterized, the visual field changes. This dynamic, unpredictable environment necessitates the ability to continuously monitor and understand sensory data input. We do not currently have any clinically-approved robotic platforms that have the ability to sense the soft tissue environment in real-time.
Planning is the second capability required for robotic surgical autonomy. Generation of a plan means that the objectives of a task are defined, and a sequence of movements can be developed to achieve those objectives. Planning also involves making decisions based on the “sensing” information gathered about the surgical environment. Importantly, full autonomy would require that the robot be able to adjust the plan along the way, in real-time.
In the research setting, motion planning is currently being applied to sub-tasks like suturing, knot tying, surgical cutting, and debridement (13,18-22). There has been recent experience with more complex tasks such as intestinal anastomosis (23). In the operative setting, autonomous and semi-autonomous platforms are limited predominantly to bony procedures. The RoboDoc (Integrated Surgical Systems) system is used in joint replacement surgery and allows the surgeon to select an implant model and specify the desired position of the implant relative to CT coordinates. Intraoperatively, the robot is registered to the patient and then it plans and cuts the exact desired shape of the bone for implant placement (24). Similarly, robots have been used for autonomous drilling in skull base surgery, cortical mastoidectomies and cochlear implantation (25-30).
At present, the planning of basic tasks is well within our capabilities. More complex tasks can be performed by breaking the task down into steps, sub-steps, and individual motions to making automating surgical tasks more realistic (31). While surgical tasks can be broken into finite movements, the combinations of those movements for specific tasks in individual surgical environments are infinite. Training a computer to assemble the proper combination of sub-steps for the ultimate completion of an entire surgery is a formidable challenge. For the development of clinical surgical autonomy, it may be most feasible to start with very basic (non-critical) sub-tasks, and gradually progress in complexity as technology and regulations permit.
After planning is complete, the robot must physically act and execute the plan—the action stage. The robot needs to know how to move, and how to alter movements if the environment changes or if a desired action does not result in the intended (or predicted) effect. The simplest form of surgical automation is execution of predetermined steps in a controlled environment. This can be likened to an assembly line robot (automaton) that carries out its task efficiently and precisely. This works well in a controlled (constrained) environment like an assembly line, but does not translate to a complex dynamic operative environment. In surgery, a predefined action sequence will fail if there is unplanned movement or deformation in the surgical field. Thus, in a dynamic surgical environment, an autonomous robot must continually process the sensing, planning, and execution stages to constantly adapt in order to perform surgical tasks precisely, effectively, and safely.
Some semi-autonomous technologies utilize virtual fixtures, a type of software program that incorporates sensing and alterations in motion plan and execution. Virtual fixtures can be thought of as “no fly zones” where motion can be prevented. An autonomous algorithm can be envisioned that integrates real-time perception to prevent damage to vital structures such as nerves or blood vessels. Virtual fixtures can also be used to alter motion within a certain three-dimensional space. In certain parts of the operative field, the robot could follow predetermined motion paths, guide the instruments along ideal trajectories or prevent the robot from entering restricted regions (12,32). Motion scaling and tremor reduction are also routinely used to enhance robotic movements (33,34), and can be applied to virtual fixtures.
Potential applications of robotic autonomy in endocrine surgery
In our current surgical practice, intelligent computer assistance is an untapped yet promising realm with immense potential. Intelligent robotic systems have potential to improve accuracy and precision, safety, and decrease human error by augmenting the way the surgeon evaluates and interacts with the surgical field. Additionally, advanced surgical robots with autonomous capabilities could increase access to high-level surgical care in rural or underserved areas, and bring surgery to otherwise unreachable austere environments. While there are currently no autonomous or semi-autonomous robotic platforms in the field of endocrine surgery, we can consider how current technologies may contribute to future innovations. A discussion of current state of the art in robotic endocrine surgery is out of the scope of this review.
Robotic autonomy could be implemented through continuous optimization of instrument trajectory and position. The robot could maintain the instrument tips in the surgeon-specified (or ideal) location while moving the control arms outside of the operative field to prevent collisions and optimize the angle of approach. This semi-autonomy augments the surgeon’s capabilities and allows the surgeon to focus on the procedure itself and not the docking and setup mechanics. This may be particularly helpful for minimally-invasive thyroid and neck surgery where much of the surgeon’s mental capital is spent on optimizing exposure and altering robotic arm trajectory and alignment.
Intelligent sensing technologies could augment the robotic endocrine surgeon’s understanding of the operative field and could be used to add safety measures such as virtual fixtures. Virtual fixtures can be thought of as regions within the operative field where robotic motion can be adjusted by the robot. This could be done to prevent motion into a carotid artery, or to automatically increase the precision and minimize force during the dissection of structures like laryngeal nerves.
The use of near-infrared fluorescence (NIRF) technology is well described in endocrine surgery. Open field fluorescence imaging, most commonly through the use of indocyanine green, is used for intraoperative identification of hyperplastic parathyroid glands and ectopic parathyroid glands in parathyroid surgery, and, in some cases, healthy parathyroid glands during thyroid operations (35,36). A label-free, real-time technique using near-infrared autofluorescence for parathyroid localization has also been described (37). Fluorescent technology has also been utilized for oncologic purposes; fluorescent labeling of peptides has been used for detection of residual papillary thyroid carcinoma (38).
The most recent da Vinci robotic endoscopes have been redesigned with an integrated fluorescent imaging mode. Early work with NIRF imaging in robotic surgery has shown promise for semi-autonomous soft tissue tracking (17,23). The near-infrared spectrum allows for tissue penetration through layers of obstructing blood or tissue (17). NIRF imaging may have clinical applications in lymph node mapping, tumor mapping, and visualization of surgical structures (39). Merging robotic NIRF technologies for visualization and intraoperative fluorescent imaging for parathyroid or tumor localization is a logical next step in the evolution of robotic endocrine surgery.
Improved surgical visualization may come through fluorescence imaging of nerves (40), gland tissue, and cancer (41). This anatomical detail could be overlaid on the surgical scene to augment traditional white light reflectance images. Even more advanced would be to have the robotic system display deeper structures as phantoms under the surface anatomy, to provide the surgeon with a window into the tissues beyond current capabilities. This improved sensing and perception could then be utilized in the next step, altering and developing semi-autonomous or autonomous motion planning and execution.
Endocrine surgery may be an attractive space for future robotic semi-autonomy research due to high disease prevalence and surgical volume and the field’s relative familiarity with fluorescence imaging. Through the complex integration of advanced imaging and control systems, a surgical robot could theoretically perform fully-autonomous parathyroidectomy, thyroidectomy, and neck dissection. We are unlikely to see this level of sophistication in our current era of endocrine surgery, but the fundamental engineering and translational research toward such sophistication will continue.
Conclusions
In 2019, replacing a surgeon with a robot remains a far-fetched concept. However, we foresee a future where surgeon-capabilities are augmented by autonomous robots—not supplanted by them. There are currently no approved applications of robotic autonomy being used in the field of endocrine surgery, but exciting emerging technologies are likely to change the way we think and operate. The gradual integration of semi-autonomous capabilities may enhance operations in the next-wave of surgical robotics. Fluorescence imaging may be a stepping stone for the development of platforms with the sensory capabilities required for designing autonomous behaviors.
Acknowledgments
Funding: None.
Footnote
Provenance and Peer Review: This article was commissioned by the Guest Editors (Jonathon Russell and Jeremy Richmon) for the focused issue “The Management of Thyroid Tumors in 2021 and Beyond” published in Annals of Thyroid. The article has undergone external peer review.
Conflicts of Interest: All authors have completed the ICMJE uniform disclosure form (available at http://dx.doi.org/10.21037/aot-19-53). The series “The Management of Thyroid Tumors in 2021 and Beyond” was commissioned by the editorial office without any funding or sponsorship. EKF reports grants from NIH/NIDCD, during the conduct of the study. FR reports non-financial support from Intuitive Surgical, during the conduct of the study; in addition, FR has a patent Motion scaling for time delayed robotic surgery pending, and a patent Augmented reality for time delayed telsurgical robotics pending. MCY reports non-financial support from Intuitive Surgical, during the conduct of the study; In addition, MCY has a patent Motion scaling for time delayed robotic surgery pending, and a patent Augmented reality for time delayed telsurgical robotics pending. RKO reports non-financial support from Intuitive Surgical, during the conduct of the study; In addition, RKO has a patent Motion scaling for time delayed robotic surgery pending, and a patent Augmented reality for time delayed telsurgical robotics pending. WSP has no other conflicts of interest to declare.
Ethical Statement: The authors are accountable for all aspects of the work in ensuring that questions related to the accuracy or integrity of any part of the work are appropriately investigated and resolved.
Open Access Statement: This is an Open Access article distributed in accordance with the Creative Commons Attribution-NonCommercial-NoDerivs 4.0 International License (CC BY-NC-ND 4.0), which permits the non-commercial replication and distribution of the article with the strict proviso that no changes or edits are made and the original work is properly cited (including links to both the formal publication through the relevant DOI and the license). See: https://creativecommons.org/licenses/by-nc-nd/4.0/.
References
- Terris DJ, Singer MC, Seybt MW. Robotic facelift thyroidectomy: patient selection and technical considerations. Surg Laparosc Endosc Percutan Tech 2011;21:237-42. [Crossref] [PubMed]
- Byeon HK, Holsinger FC, Duvvuri U, et al. Recent progress of retroauricular robotic thyroidectomy with the new surgical robotic system. Laryngoscope 2018;128:1730-7. [Crossref] [PubMed]
- Liu P, Zhang Y, Qi X, et al. Unilateral Axilla-Bilateral Areola Approach for Thyroidectomy by da Vinci Robot: 500 Cases Treated by the Same Surgeon. J Cancer 2019;10:3851-9. [Crossref] [PubMed]
- Prete FP, Marzaioli R, Lattarulo S, et al. Transaxillary robotic-assisted thyroid surgery: technique and results of a preliminary experience on the Da Vinci Xi platform. BMC Surg 2019;18:19. [Crossref] [PubMed]
- Piccoli M, Mullineris B, Gozzo D, et al. Evolution Strategies in Transaxillary Robotic Thyroidectomy: Considerations on the First 449 Cases Performed. J Laparoendosc Adv Surg Tech A 2019;29:433-40. [Crossref] [PubMed]
- Lee KE, Rao J, Youn YK. Endoscopic thyroidectomy with the da Vinci robot system using the bilateral axillary breast approach (BABA) technique: our initial experience. Surg Laparosc Endosc Percutan Tech 2009;19:e71-75. [Crossref] [PubMed]
- Cho JN, Park WS, Min SY, et al. Surgical outcomes of robotic thyroidectomy vs. conventional open thyroidectomy for papillary thyroid carcinoma. World J Surg Oncol 2016;14:181. [Crossref] [PubMed]
- Lee HY, You JY, Woo SU, et al. Transoral periosteal thyroidectomy: cadaver to human. Surg Endosc 2015;29:898-904. [Crossref] [PubMed]
- Richmon JD, Kim HY. Transoral robotic thyroidectomy (TORT): procedures and outcomes. Gland Surg 2017;6:285-9. [Crossref] [PubMed]
- Cottrill EE, Funk EK, Goldenberg D, et al. Transoral Thyroidectomy Using A Flexible Robotic System: A Preclinical Cadaver Feasibility Study. Laryngoscope 2019;129:1482-7. [Crossref] [PubMed]
- Kim HK, Chai YJ, Dionigi G, et al. Transoral Robotic Thyroidectomy for Papillary Thyroid Carcinoma: Perioperative Outcomes of 100 Consecutive Patients. World J Surg 2019;43:1038-46. [Crossref] [PubMed]
- Yip M, Das N. Robot Autonomy for Surgery. arXiv:1707.03080.
- Taylor R, Jensen P, Whitcomb L, et al. A Steady-Hand Robotic System for Microsurgical Augmentation. Berlin, Heidelberg: Springer Berlin Heidelberg, 1999;1031-41.
- Kapoor A, Kumar R, Taylor RH. Simple Biomanipulation Tasks with “Steady Hand” Cooperative Manipulator. In. Berlin, Heidelberg: Springer Berlin Heidelberg 2003;141-8.
- Schweikard A, Tombropoulos R, Kavraki L, et al. Treatment planning for a radiosurgical system with general kinematics. In Proceedings of the 1994 IEEE International Conference on Robotics and Automation 1994. DOI:
10.1109/ROBOT.1994.351344 . - Bouget D, Allan M, Stoyanov D, et al. Vision-based and marker-less surgical tool detection and tracking: a review of the literature. Med Image Anal 2017;35:633-54. [Crossref] [PubMed]
- Shademan A, Dumont MF, Leonard S, et al. Feasibility of near-infrared markers for guiding surgical robots. SPIE, 2013.
- Murali A, Sen S, Kehoe B, et al. Learning by observation for surgical subtasks: Multilateral cutting of 3D viscoelastic and 2D Orthotropic Tissue Phantoms. In 2015 IEEE International Conference on Robotics and Automation (ICRA) 2015:1202-9.
- Thananjeyan B, Garg A, Krishnan S, et al. Multilateral surgical pattern cutting in 2D orthotropic gauze with deep reinforcement learning policies for tensioning. In 2017 IEEE International Conference on Robotics and Automation (ICRA) 2017:2371-8.
- Kehoe B, Kahn G, Mahler J, et al. Autonomous multilateral debridement with the Raven surgical robot. In 2014 IEEE International Conference on Robotics and Automation (ICRA) 2014:1432-9.
- Leonard S, Wu KL, Kim Y, et al. Smart tissue anastomosis robot (STAR): a vision-guided robotics system for laparoscopic suturing. IEEE Trans Biomed Eng 2014;61:1305-17. [Crossref] [PubMed]
- Hyosig K, Wen JT. Autonomous suturing using minimally invasive surgical robots. In Proceedings of the 2000. IEEE International Conference on Control Applications. Conference Proceedings (Cat. No.00CH37162) 2000:742-7.
- Shademan A, Decker RS, Opfermann JD, et al. Supervised autonomous robotic soft tissue surgery. Sci Transl Med 2016;8:337ra64. [Crossref] [PubMed]
- Taylor RH, Mittelstadt BD, Paul HA, et al. An image-directed robotic system for precise orthopaedic surgery. IEEE Transactions on Robotics and Automation 1994;10:261-75. [Crossref]
- Brett PN, Taylor RP, Proops D, et al. A surgical robot for cochleostomy. Conf Proc IEEE Eng Med Biol Soc 2007;2007:1229-32. [Crossref] [PubMed]
- Coulson CJ, Reid AP, Proops DW. A cochlear implantation robot in surgical practice. In 2008 15th International Conference on Mechatronics and Machine Vision in Practice 2008;173-6.
- Coulson CJ, Taylor RP, Reid AP, et al. An autonomous surgical robot for drilling a cochleostomy: preliminary porcine trial. Clin Otolaryngol 2008;33:343-7. [Crossref] [PubMed]
- Hussong A, Rau TS, Ortmaier T, et al. An automated insertion tool for cochlear implants: another step towards atraumatic cochlear implant surgery. Int J Comput Assist Radiol Surg 2010;5:163-71. [Crossref] [PubMed]
- Majdani O, Rau TS, Baron S, et al. A robot-guided minimally invasive approach for cochlear implant surgery: preliminary results of a temporal bone study. Int J Comput Assist Radiol Surg 2009;4:475-86. [Crossref] [PubMed]
- Xia T, Baird C, Jallo G, et al. An integrated system for planning, navigation and robotic assistance for skull base surgery. Int J Med Robot 2008;4:321-30. [Crossref] [PubMed]
- Berg Jvd, Miller S, Duckworth D, et al. Superhuman performance of surgical tasks by robots using iterative learning from human-guided demonstrations. In 2010 IEEE International Conference on Robotics and Automation 2010:2074-81.
- Selvaggio M, Fontanelli GA, Ficuciello F, et al. Passive Virtual Fixtures Adaptation in Minimally Invasive Robotic Surgery. IEEE Robotics and Automation Letters 2018;3:3129-36. [Crossref]
- Leal Ghezzi T, Campos Corleta O. 30 Years of Robotic Surgery. World J Surg 2016;40:2550-7. [Crossref] [PubMed]
- Richter F, Orosco RK, Yip MC. Motion Scaling Solutions for Improved Performance in High Delay Surgical Teleoperation. In 2019 International Conference on Robotics and Automation (ICRA) 2019;1590-5.
- Fanaropoulou NM, Chorti A, Markakis M, et al. The use of Indocyanine green in endocrine surgery of the neck: A systematic review. Medicine (Baltimore) 2019;98:e14765. [Crossref] [PubMed]
- van den Bos J, van Kooten L, Engelen SME, et al. Feasibility of indocyanine green fluorescence imaging for intraoperative identification of parathyroid glands during thyroid surgery. Head Neck 2019;41:340-8. [PubMed]
- McWade MA, Paras C, White LM, et al. Label-free intraoperative parathyroid localization with near-infrared autofluorescence imaging. J Clin Endocrinol Metab 2014;99:4574-80. [Crossref] [PubMed]
- Orosco RK, Savariar EN, Weissbrod PA, et al. Molecular targeting of papillary thyroid carcinoma with fluorescently labeled ratiometric activatable cell penetrating peptides in a transgenic murine model. J Surg Oncol 2016;113:138-43. [Crossref] [PubMed]
- Tummers QRJG, Schepers A, Hamming JF, et al. Intraoperative guidance in parathyroid surgery using near-infrared fluorescence imaging and low-dose Methylene Blue. Surgery 2015;158:1323-30. [Crossref] [PubMed]
- Walsh EM, Cole D, Tipirneni KE, et al. Fluorescence imaging of nerves during surgery. Ann Surg 2019;270:69-76. [Crossref] [PubMed]
- Nguyen QT, Tsien RY. Fluorescence-guided surgery with live molecular navigation--a new cutting edge. Nat Rev Cancer 2013;13:653-62. [Crossref] [PubMed]
Cite this article as: Funk EK, Richter F, Park WS, Yip MC, Orosco RK. Robotic autonomy in endocrine surgery. Ann Thyroid 2020;5:7.